PerMonLiSt
Continuous Performance Monitoring and Calibration of Model and Control Functions for Liquid Steelmaking Processes
PerMonLiSt project objectives
The main objective of the research project was to improve, for the different stages of the liquid steelmaking process route, the continuous monitoring of the process performance as well as to ensure the permanent reliability of used dynamic process models and control rules. For this purpose, methods and tools have been developed involving the application of innovative and comprehensive performance indexes and strategies for automatic calibration of model and control parameters.
By these developments the following benefits should be achieved for the liquid steelmaking processes:
- Improved on-line monitoring of the process performances, to be used by engineers and operators to decide about necessary countermeasures. Moreover, the increased knowledge about the process behaviour can be used to improve the operating practices.
- Long-term reliable operation of dynamic process models and rule based set-point calculations used for off-line process optimisation as well as on-line monitoring and process control, by continuous monitoring of model and control performance with automatic adaptation of related parameters – e.g. by least-squares-fitting, Kalman filter and machine learning approaches.
- Improved reliability and stability of the liquid steelmaking processes by enhanced performance of model- and rule-based control of analysis and temperature of the steel melt with reduced scatter and deviations from the desired target values.
- Minimisation of energy and resources consumption as well as treatment duration by enhanced reliability of level-2 automation and process control functions.
The developed tools have been coupled to an integrated approach and tested exemplarily for the most important liquid steelmaking facilities of the electric steelmaking route, i.e. for electrical arc furnace (EAF), ladle furnace (L)F, vacuum degasser (VD) and argon stirring plants.
The project started at July 1st 2016 and ended at December 31st 2019. Involved partners in the research were:
VDEH-Betriebsforschungsinsitut GmbH |
  |
Centre for Research in Metallurgy |
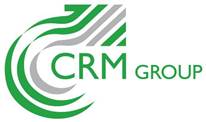 |
Feralpi Siderurgica S.p.A. |
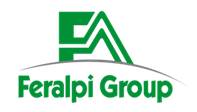 |
Centro Sviluppo Materiali |
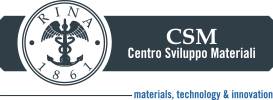 |
Peiner Träger GmbH |
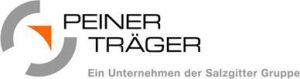 |
Horizon 2020 |
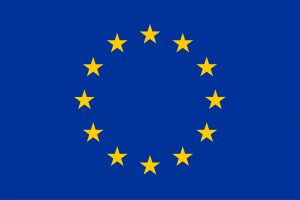 |
The research has received funding from the European Union’s Research fund for Coal and Steel (RFCS) under grant agreement No. RFSR-CT-2016-709620.
PerMonLiSt achieved results
In a first step, the available process models and required process data have been described and assessed regarding current accuracies for the EAF and secondary metallurgical ladle treatment processes at PTG, Feralpi/Lonato and Tata/Aldwarke, respectively. Data acquisition and model functions for EAF and ladle treatment processes at PTG as well as EAF process at Feralpi/Lonato and Tata/Aldwarke steel plant have been completed.
Process and model performance indexes have been defined for assessment of process behaviour and related model calculations in electric steelmaking processes. The analysed correlations between process performance indexes and operating practices show different significances. The most significant correlation for all studied EAFs of the industrial partners is given between metallic yield and specific oxygen consumption (see Figure A). The relation of specific energy consumption decreasing with increasing productivity in EAF depends on the characteristics of the furnace and its operation. The desulphurisation efficiency in ladle treatment shows positive correlation with the volume of applied stirring gas. Such correlations were used to appropriately adapt related operating practices. Analysed correlations between model and process performance indexes reveal systematic errors of the respective model for certain ranges of process operation.
Figure A: Correlation between specific O2 gas consumption and metallic losses for different EAFs of industrial partners
Regular ranges for defined process performance indices have been defined which are used within enhanced monitoring and alert functions regarding process behaviour and performances. At Feralpi Lonato steel plant such functions have been included in the newly installed on-line system EAFPro covering the EAF as well as the subsequent ladle treatment processes until teeming in tundish. The implemented monitoring functions comprise also new control charts with statistical evaluations of relevant process parameters and performance indices. Regarding enhancement of process monitoring, PTG has laid the focus on the EAF process. A suitable new human machine interface (HMI) has been designed and installed within their manufacturing execution system (MES) to support the operator in end-point control of the EAF process (see below). Furthermore, operating practices for EAF and secondary metallurgy have been adapted via appropriate configuration of the MES. For the secondary metallurgical ladle treatment, the specification of target temperature and time for delivery of a heat to the caster has been extended to take into account the position of the heat in the casting sequence. This information is used for dynamic adaptions of defined variable set-points in the operating practices (e.g. electrical energy input in LF) based on predictive model calculations.
The operating instructions at Feralpi Lonato plant have been adapted regarding practices with charging of 2 or 3 scrap baskets, charging and injection of carbon as well as injection of oxygen in the EAF. Charging of 2 scrap baskets results in lower electrical energy consumption for cases with sufficiently high scrap densities. The charging and injection of carbon is reduced in cases of too high carbon contents analysed in LF for previous heats. Additional increase of injected oxygen during EAF refining phase reduces carbon content further on with lowering electrical energy consumption and tap to tap time but also with decrease of metallic yield. The management of optimised oxygen injection as well as charge and injection of carbon in order to achieve maximum metallic yield, target carbon content in steel and sufficient slag foaming in EAF is supported by related control rules. Figure B shows an example for control of oxygen injection using the calculated slag oxidation status (SOS = calculated slag weight / reference slag weight) and appropriate thresholds for adaption of the injection practice (based on reference slag weight of 5 t).
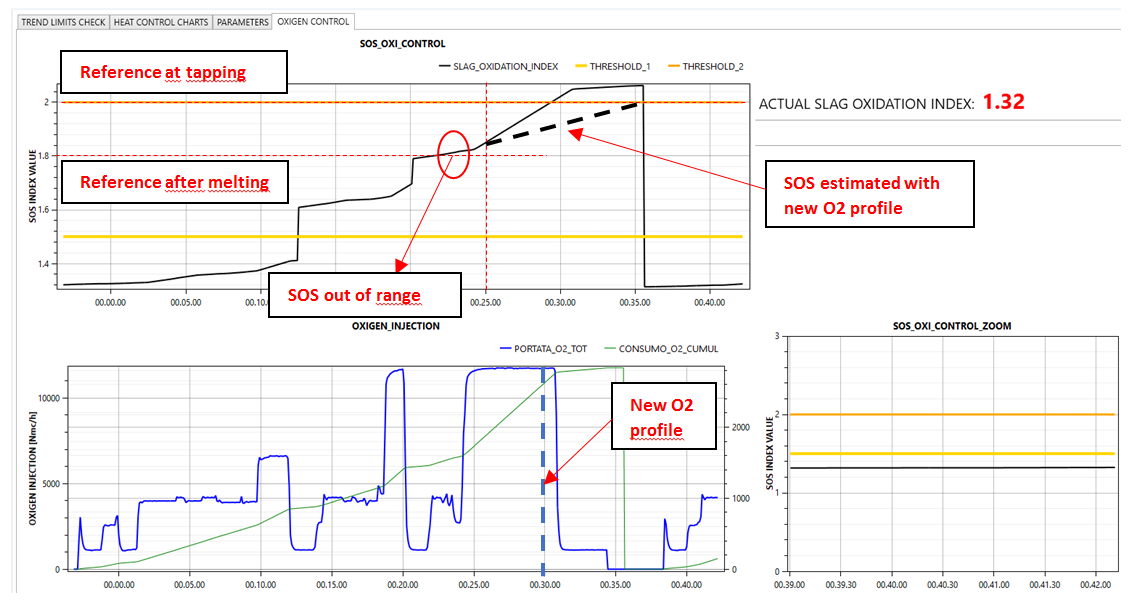
Figure B: Logics of control function implemented at Feralpi Lonato for adaption of oxygen injection using slag oxidation status (SOS)
For applications with large number of model and control parameters, auto-calibration methods based on regression analysis with least-squares fitting and artificial neural network have been chosen, while approaches using Unscented Kalman filters (UKF), moving average corrections or KPI (key performance index) based heuristics were found to be suitable for on-line heat-to-heat adaptions of few parameters with rare measurements.
BFI has set-up an off-line calibration procedure for the parameters of the dynamic EAF model installed at PTG furnaces based on regression analysis with least-squares fitting on a defined number (e.g. 200) of last produced heats. This procedure can be called after a certain number (e.g. 100) of newly produced heats in order to keep the parameters adapted to the respective scrap, plant and process conditions. For the secondary metallurgical temperature model an on-line batch-to-batch adaption of identified (significant) parameters distinguished for 3 different routes (with 3 different LFs) has been developed using an Unscented Kalman filter (UKF) approach. Figure C exemplarily shows the resulting evolution of the electrical energy efficiency for one ladle furnace with an initial decrease of this efficiency which is later recovered again.
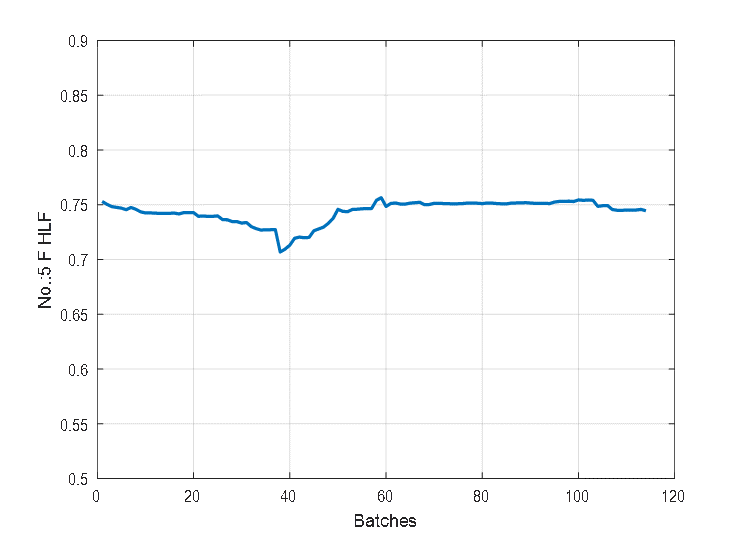
Figure C: Batch-to-batch adaption of electrical energy efficiency at ladle furnace of PTG steel plant
CRM has set-up a parameter calibration for their EAF model based on the same Kalman filter technique. It is important to select the most significant parameters and to adapt not more parameters in one step than the available number of temperature measurements for the related heat. The convergence speed of the Kalman filter should be adjusted by appropriate selection of the covariance matrix of applied process noise, so that the parameters converge on average over 10 -20 adaption steps.
CSM and Feralpi have developed an artificial neural network solution (ANN) for auto-calibration of the scrap basket composition at Feralpi Lonato plant (see Figure D). The sterile content of scrap also has to be taken into account in the slag weight which is estimated by the used EAFPro model to assess the slag oxidation status (SOS). This is a KPI mainly used at Feralpi Lonato plant within control rules for oxygen injection during the EAF refining phase (cf. Figure B). Another developed method for auto-calibration of the sterile content in scrap uses a moving average over the difference between calculated and measured tap weights of steel.
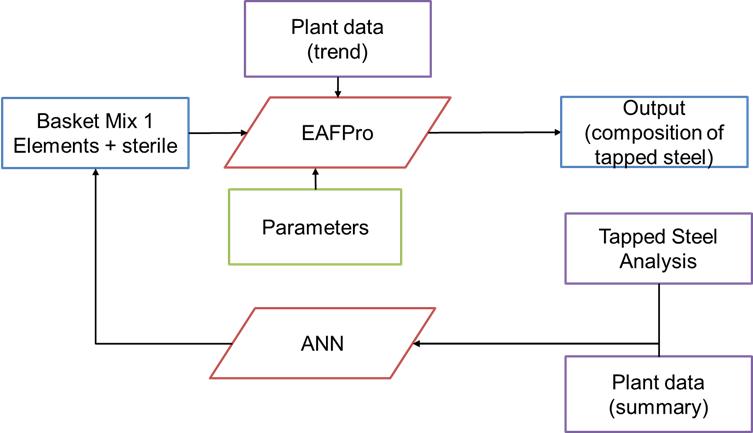
Figure D: Auto-calibration loop for scrap basket composition at Feralpi Lonato plant using an ANN
Furthermore, a temperature model for ladle treatment between tapping at EAF and teeming in tundish has been set-up by CSM and Feralpi which is also based on a calibration of model parameters via statistical analysis with moving average adaptions. The auto-calibrated model calculations are used for predictions of arrival temperatures in LF and CC. Together with defined acceptable limits for these arrival temperatures, the model predictions are used to support the operator in control of the thermal state evolution along the whole electric steelmaking route. This kind of temperature control together with the already mentioned adaptions of oxygen injection as well as carbon charge and injection based on evaluation of slag foaming via an acoustic sensor in EAF build an expert system of control rules for process management at Feralpi Lonato plant. For the used control rule parameters (e.g. limits for SOS and acoustic foaming index) there have been derived heuristics based on related KPIs for suitable auto-calibrations. For the auto-calibration of the SOS limits used within the control rules for oxygen injection (which were in the main focus for the EAF process at Feralpi Lonato), the heuristic uses information from the adapted sterile content in scrap.
At PTG as well as at Feralpi Lonato steel plant the installed tools support the operator in on-line monitoring and control of the EAF and secondary metallurgical ladle treatment processes, especially regarding electrical and chemical heating. Figure E gives an example of the newly installed HMI for online monitoring of the EAF processes at PTG steel plant. For support of end-point control of the heating process, the horizontal red line in the upper half indicates the target tapping temperature to be met by the actual temperature calculated cyclically by the EAF model.
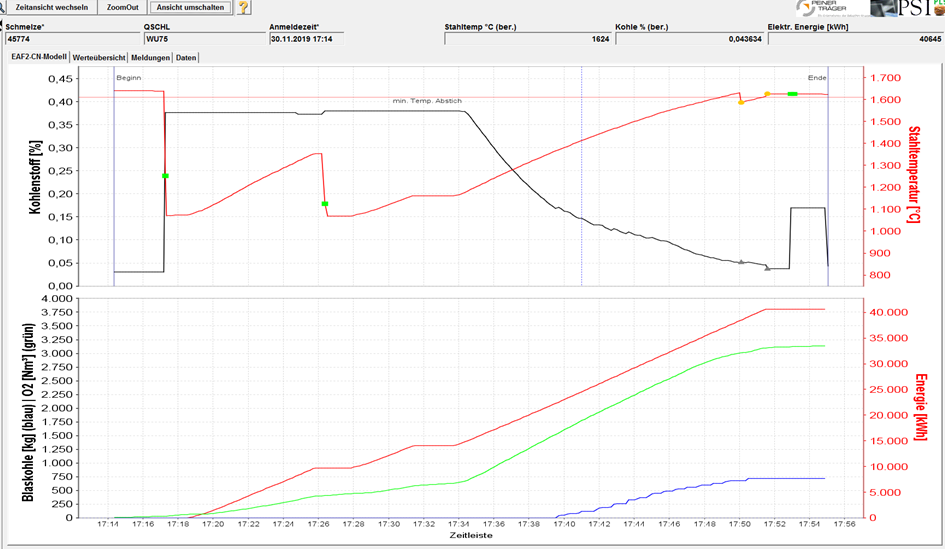
Figure E: HMI for online monitoring and control of EAF processes at PTG steel plant
The installed least-squares fitting procedure for periodic adaption of the EAF model parameters mainly improves the mean model error (from mainly negative values to values around 0), whereas the standard deviation of the model error more or less is unaffected (cf. Figure F). The improvement of the mean model error results in an avoidance of systematic over- or underestimation of the melt temperature by about 5 – 10 K. Cases with systematic underestimation as shown in Figure F cause an overheating of the melt with related increase of
- specific electrical energy consumption by about 3.5 – 7 kWh/t
- and of tap-to-tap times by about 15 – 30 s
as well as negative effects on the lifetime of the furnace refractory. Cases with systematic overestimation of the melt temperature lead to need of
- further heating in EAF after a temperature measurement with related increase of tap-to-tap times
- or additional heating in LF (if such a measurement is not taken before tapping) with related longer treatment times and thereby lower productivity.
Figure F: Evolution of moving averages and standard deviations of errors in model temperatures at PTG with and without recalibration of parameters at the DC-EAF
The secondary metallurgical temperature model installed at PTG steel plant turned out to be already well calibrated and quite stable regarding the plant and process conditions. Thus, the batch-to-batch auto-calibration procedure based on the UKF approach has been tested after detuning of model parameters. This proved an automatic re-calibration of the model by the installed UKF procedure within about 20 heats resulting in reduced mean values and standard deviations of the model errors compared to the detuned cases.
The off-line application of the UKF approach for batch-to-batch auto-calibration of the CRM EAF model provided significant improvements of calculation accuracies (see Figure G). The mean error is decreased from 18,7 K to 3,5 K and the standard deviation from 58,8 K to 23,1 K.
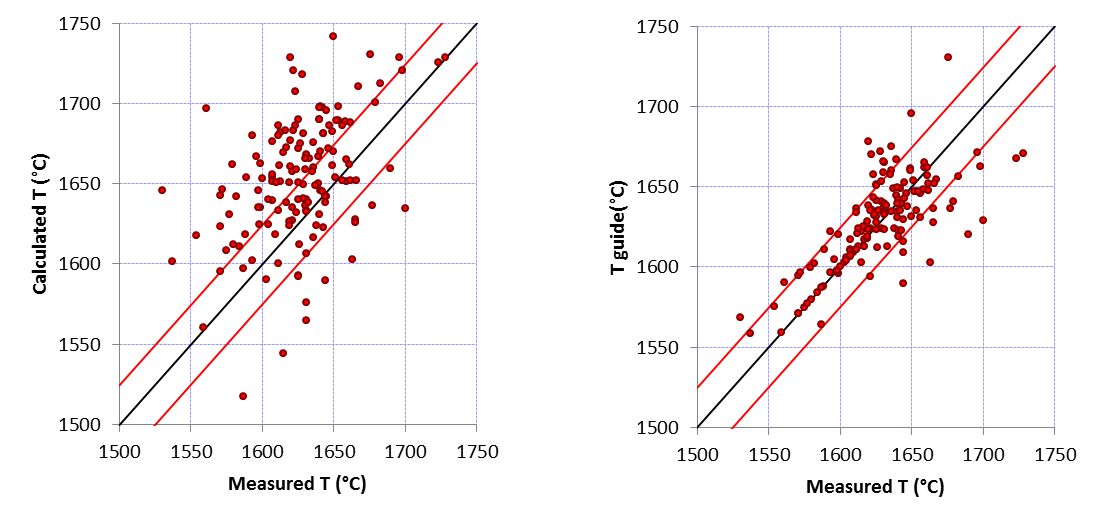
Figure G: Correlation between calculated and measured temperature without (left) and with (right) auto-tuning of parameters for CRM EAF model
Thus, the on-line application of the CRM EAF model with batch-to-batch auto-calibration and thereby ensured improved model accuracies will lead to similar benefits as estimated above for the BFI EAF model applied at PTG steel plant.
For improved process monitoring and control of the EAF process at Feralpi Lonato steel plant, the auto-calibration of sterile content in scrap has been identified as one of the most important components. A moving average adaption of this parameter yields improved results for mass balance calculations including slag oxidation status (SOS) used for control of oxygen injection. Figure H gives an example for monitoring of the auto-calibrated sterile content of scrap and the related steel weight error.
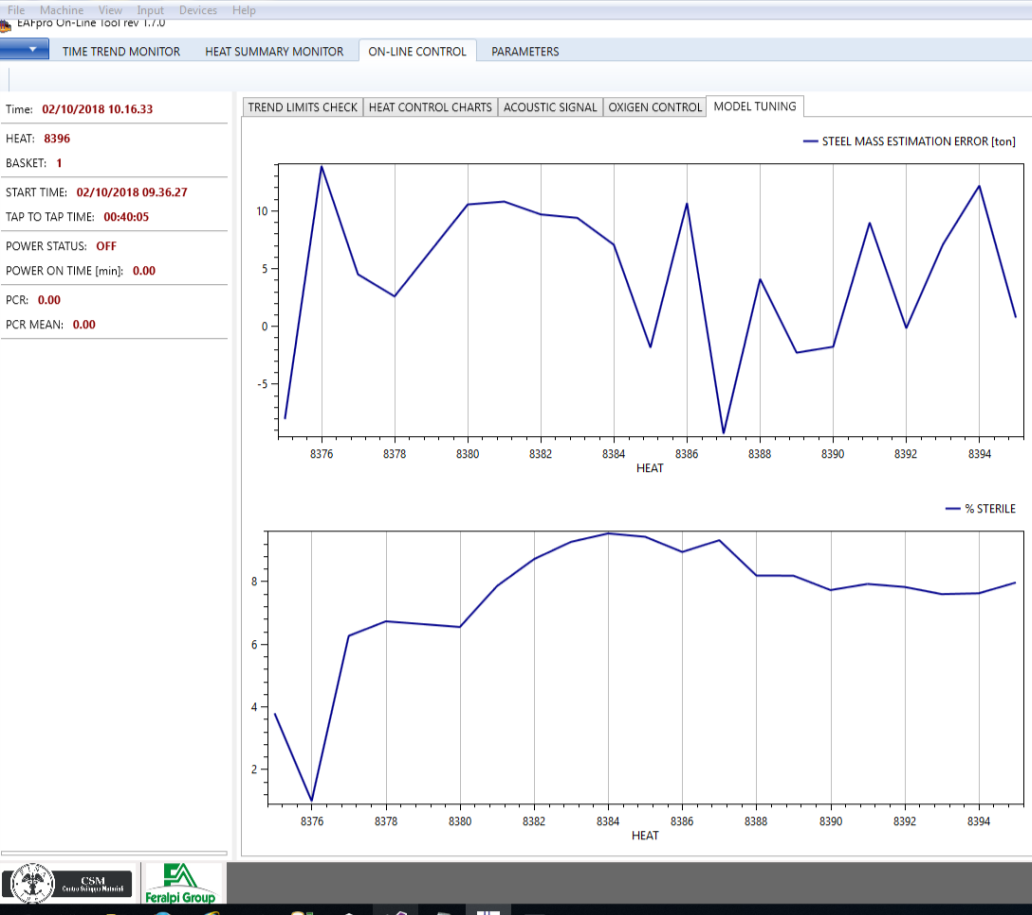
Figure H: Monitoring of auto-calibrated sterile content of scrap and related steel weight error
The SOS based expert rules for on-line control of oxygen injection during refining in EAF lead to avoidance of overoxidation with reduced consumptions of electrical energy and oxygen and increased metallic yield. The on average achieved improvements at Feralpi Lonato plant comprise
- reduction of specific electrical energy: 10 kWh/ton
- reduction of SOS value at end of heat: 0,02
- reduction of used oxygen volume: 250 Nm³
- increase of tap steel weight: 500 kg
- increase of metallic yield: 0,5 %
Furthermore, the rules for alerts regarding arrival temperatures in LF and CC based on the installed temperature model for ladle treatment at Feralpi Lonato plant with adapted parameters have been proven to support the operator decisions for temperature control along the whole steelmaking route.